- Home
- About
- People
- Research
- Future Students
- Current Students
- Community
- News
- Events
News
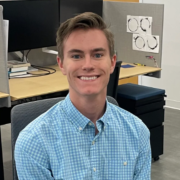
April 15, 2024
CS Student Named to the NSF Graduate Research Fellowship Program
Senior computer science Student Griffin J. Hurt has been named a 2024 Graduate Research Fellowship Program (GRFP) scholar.
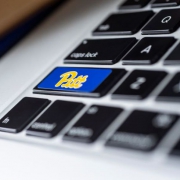
March 27, 2024
SCI Projects Awarded 2023-24 Pitt Momentum Funds
This year, three projects by faculty members at the School of Computing and Information (SCI) have received Momentum Funds from the University of Pittsburgh Office of Research.

March 26, 2024
April 19 Colloquium: "The Many Facets of the Quantum Adiabatic Theorem"
In this talk, we will discuss the evolution of this theorem and the surprising developments of the 1980s that led to the concept of the geometric phase. We will use simple models to demonstrate how QAC is applied in applications. Additionally, we will explore how the discovery of geometric phases has led to the idea of quantum holonomic computing.
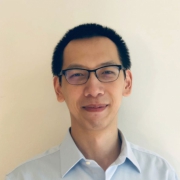
March 20, 2024
Assistant Professor Xiaowei Jia Receives the NASA Early Career Investigator Grant
Dr. Xiaowei Jia (assistant professor) received an Early Career Investigator Grant from NASA's Earth Science Division for his project “Towards Generalizable, Fair, and Knowledge Guided Machine Learning for Monitoring Earth Systems.”

March 18, 2024
April 12 Colloquium: "Learning to Understand Our Multimodal World with Minimal Supervision"
In this talk, I will present our recent research on creating AI systems that can learn to understand our multimodal world with minimal human supervision. I will focus on systems that can understand images and text, and also touch upon those that utilize video, audio, and lidar.
March 13, 2024
CS Faculty Members Receive Spring 2024 Pitt Cyber Accelerator Grants
CS Faculty Members Drs. Lorraine Li, Diane Litman, and Ryan Shi receive Spring 2024 Pitt Cyber Accelerator Grants for various projects.
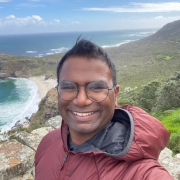
March 6, 2024
March 29 Colloquium: "Emission Factor Selection with Neural Language Models"
To avoid repetitive analysis, environmental impact factors (EIF) of common materials and products are published for use by LCA experts. However, finding appropriate EIFs for even a single product under study can require hundreds of hours of manual work, especially for complex products. We present Flamingo, an algorithm that leverages natural language machine learning (ML) models to automatically identify an appropriate EIF given a text description.
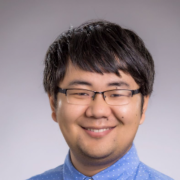
February 28, 2024
Assistant Professor Longfei Shangguan Receives an NSF CAREER Award to Democratize Earable Computing Research
Dr. Longfei Shangguan (assistant professor) received an NSF CAREER award for his project “Ubiquitous Earable Sensing Using Low-Cost Earphones.”
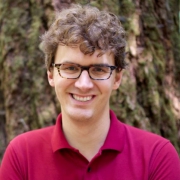
February 23, 2024
March 22 Colloquium: "Multimodal Learning from Pixels to People"
People experience the world through modalities of sight, sound, words, touch, and more. By leveraging their natural relationships and developing multimodal learning methods, my research creates artificial perception systems with diverse skills, including spatial, physical, logical, and cognitive abilities, for flexibly analyzing visual data.
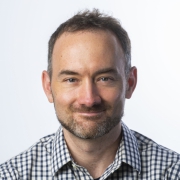
February 22, 2024
March 1 Colloquium: "Towards Automating Machine Learning Engineering"
When a skilled machine learning engineer is tasked with building a system for a specific application, they take several steps. Some of these include doing a literature review of the most appropriate models and datasets, choosing which ones to utilize based on accuracy and other constraints such as efficiency or latency, creating or curating training and testing data, training and comparing models, identifying weak points of the current modeling paradigm and iteratively improving.
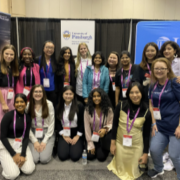
February 15, 2024
Dozens of SCI Students Attend Renowned Grace Hopper Conference
Last month, 33 students (18 in person and 15 virtually) from the School of Computing and Information (SCI) attended the Grace Hopper Conference (GHC) in Orlando, FL.
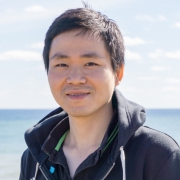
February 15, 2024
Assistant Professor Stephen Lee Receives NSF Grant for Designing Sustainable Distributed Infrastructures
Dr. Stephen Lee (assistant professor, Department of Computer Science) received a National Science Foundation (NSF) grant for his project, “Data-Driven System-Design for Sustainable Long-Lasting Distributed Infrastructures,” on Oct. 1, 2023.
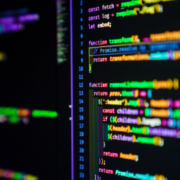
February 15, 2024
Students Create Projects Aimed at Solving Problems in Healthcare During the Pitt Challenge 2023 Hackathon
In September, students from the School of Computing and Information, along with their peers from other schools within Pitt, came together to plan the Pitt Challenge 2023.
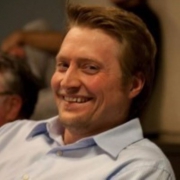
February 15, 2024
Dr. Jacob Biehl Is Building Systems That Reshape Space
For Biehl, an associate professor with a dual appointment in the Department of Information Culture and Data Stewardship and the Department of Computer Science, technical rigor and the usability needs of the people operating a system are not separate considerations.
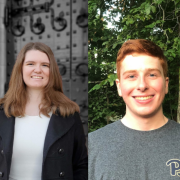
February 14, 2024
Two CS Students Receive Honorable Mention in CRA Undergraduate Research Awards
Two students from the School of Computing and Information (SCI) Department of Computer Science have been recognized for their undergraduate research by the Computing Research Association (CRA)!